You can only gain access to certain items and special pricing if you have logged in. Login Now.
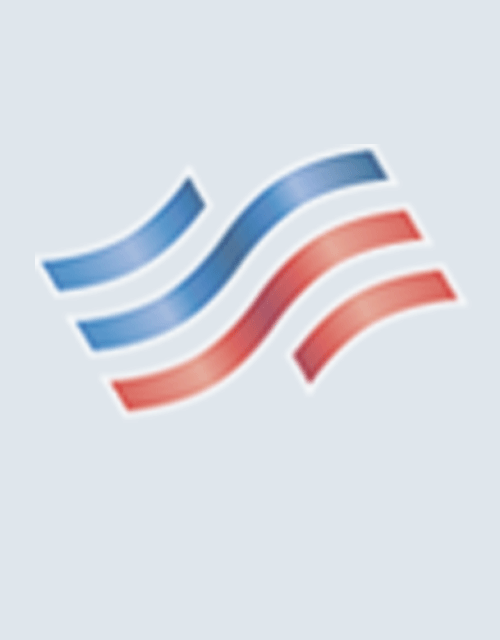
CC-96-122 HOW NEURAL NETWORK MODELING METHODS COMPLEMENT THOSE OF PHYSICAL MODELING
Warren Thompson, CITGO, Greg Martin, Pavilion Technologies, Inc.
Format:
Electronic (digital download/no shipping)
Associate Member, International Member, Petrochemical Member, Refining Member - $0.00
Government, NonMember - $35.00
Description:
The recent proliferation of neural network modeling activity in the refining and petrochemical process industries has fostered new levels of understanding about the compatibility of this empirical approach with the well-established methods of physical modeling. It has been demonstrated that the methods of large-scale neural network modeling are comnlementary with those of physical modeling in a variety of ways. Examples include modeling physical models with neural networks to increase speed of execution and reduce program size, use of neural network model information to improve physical models, and constructing large models from several individual unit or process models, for the purpose of multi-unit optimization. This paper discusses these topics, and includes examples from three industrial modeling exercises.
Product Details:
Product ID: | CC-96-122 |
---|---|
Publication Year: | 1996 |