You can only gain access to certain items and special pricing if you have logged in. Login Now.
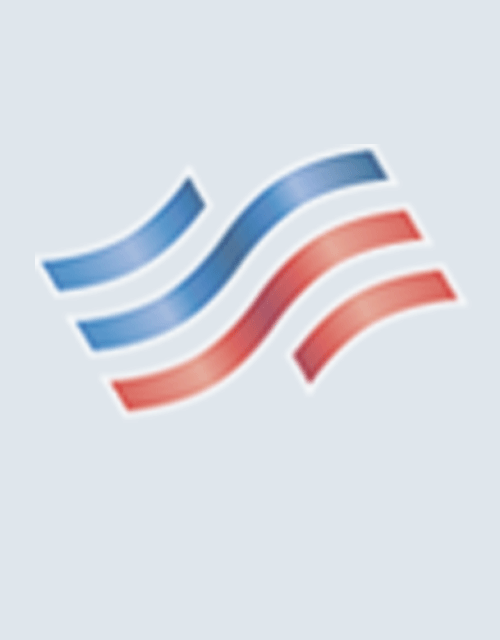
CC-02-154 DESIGNING NEURAL NETWORK GAIN MODELS THAT EXTRAPOLATE FOR NMPC
Greg Martin, Pavilion Technologies, Inc.
Format:
Electronic (digital download/no shipping)
Associate Member, International Member, Petrochemical Member, Refining Member -
$0.00
Government, NonMember -
$25.00
Description:
Historically the most well-known drawback of neural network models is that they don’t extrapolate. This paper describes a design procedure that guarantees extrapolation to whatever degree required by the application. A first-principles model is modified by a neural network to make a mixed model. This model extrapolates because it is equation-based. A neural network is trained on data produced by the mixed model. Since this data can range beyond the normal operation of the process, the neural network copy inherits extrapolation capability. A field example from polymers is provided, where nonlinearities are so severe that linear MPC cannot be tuned for satisfactory performance.
Product Details:
Product ID: | CC-02-154 |
---|---|
Publication Year: | 2002 |